Gramener and Microsoft AI for earth help
nisqually river foundation augment
fish identification by 73% accuracy
through deep learning AI models
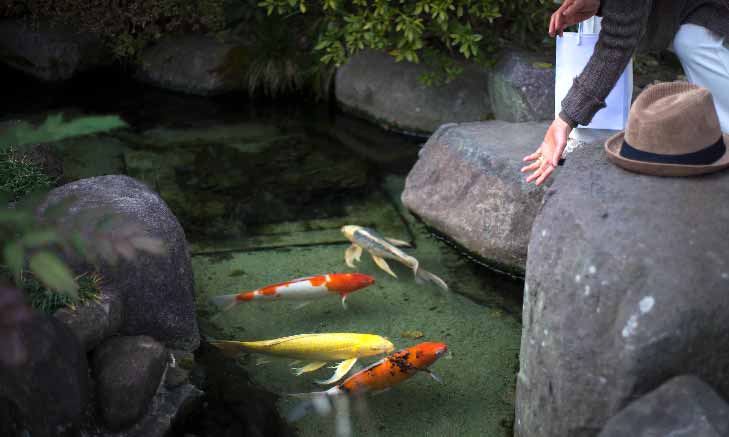
Challenge
Earlier, biologists would manually identify and record fish species
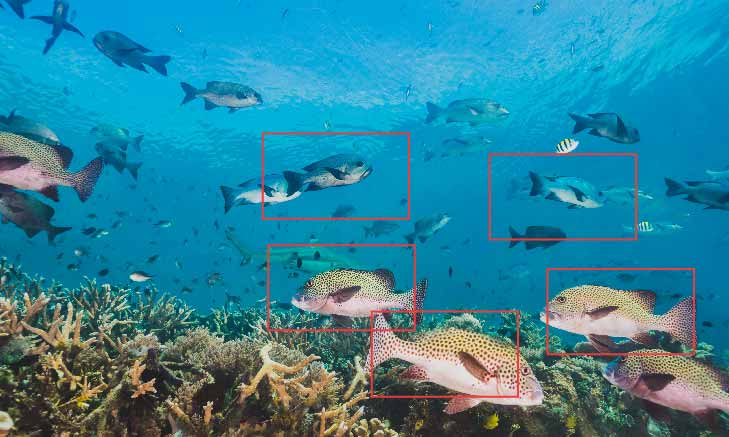
Approach
Now, Deep Learning AI models have been trained to detect the fish
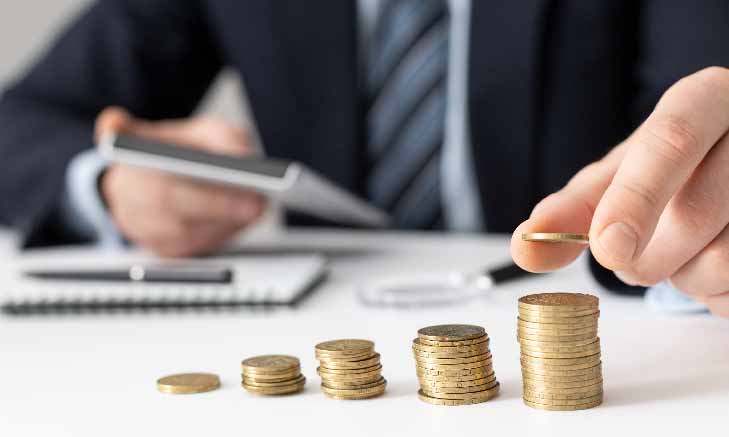
Outcome
AI-driven Image Recognition. 80% potential cost saving. Time taken reduced by 5X
A 2-minute summary of the project
THE PROBLEM
The manual process to identify and name any living species through captured videos is resource intensive from a time, human, and cost perspective. So, when the Nisqually River Foundation, a Washington based nature conservation organization, encountered a similar challenge to measure and monitor the Salmon species fish identification, they approached us for an automated technology-driven solution.
AI-DRIVEN SOLUTION FOR FISH IDENTIFICATION
First, the collected video feeds were processed to extract the relevant frames. Deep learning AI models were then trained to draw bounding boxes around each fish passing by the camera. The entire workflow encapsulated in a Web App automated the process of video feed input, detection and classification. The automated AI solution leveraged the latest implemented deep learning algorithms using the Microsoft Azure and Cognitive Services platform stack. Given the nature of the problem and the format of the video files, processing power was a key requirement for the training and validation phases. A GPU machine was the natural choice to run the object detection models. Hence, we selected the NC6 GPU VM in the Azure portal.
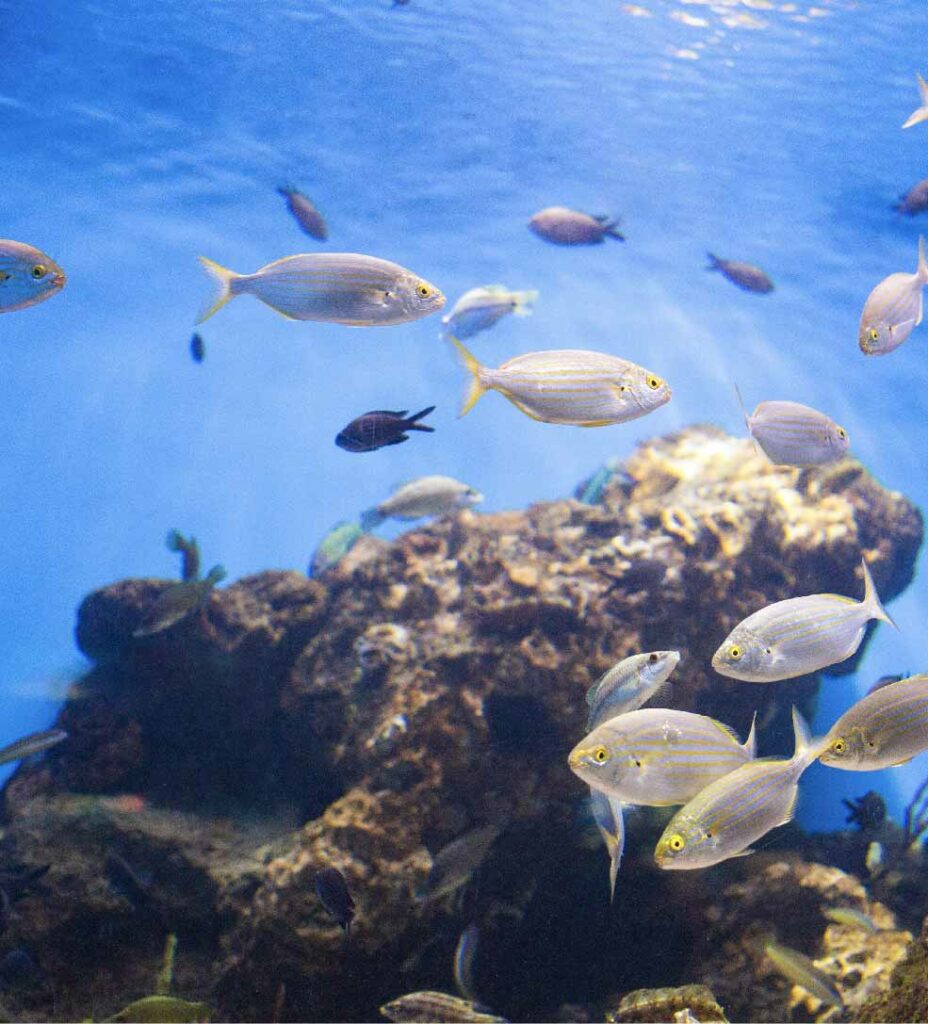
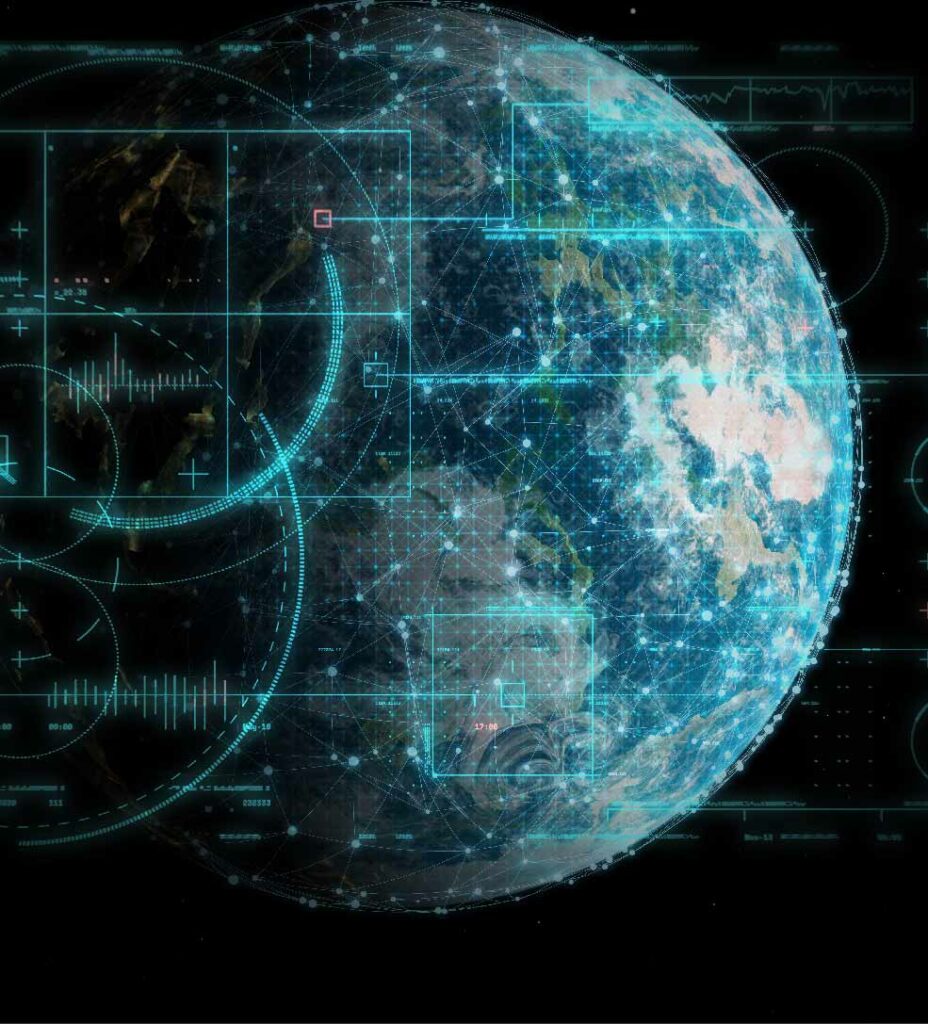
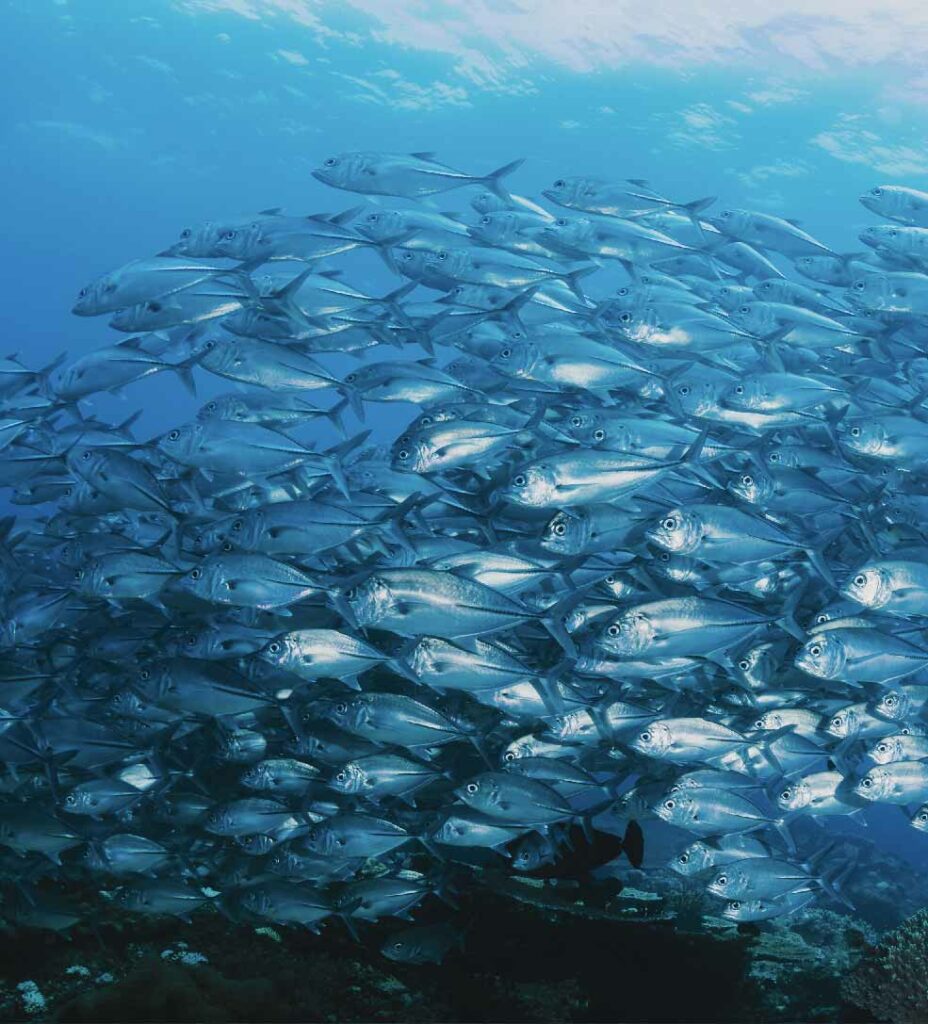
THE APPROACH
PARTNERING WITH MICROSOFT AI FOR EARTH TEAM
The Microsoft AI for Earth team was a key enabler and influencer for project’s success, through timely access for technical support and resolution of AI platform queries.
Technology Used to Resolve the Issue
- For a reliable cloud solution with machine learning capabilities, Microsoft Azure Data Science Virtual Machine (DSVM) was chosen.
- For the purpose of extracting frames from the videos and tag them, Microsoft Visual Object Tagging Tool (VOTT) came in handy.
- The final object detection algorithm chosen was the YOLO V3 Video detection algorithm.
Our Contribution to Augment the Process
- The first challenge was to process the videos and tag the fish. The heavy manual work involved in this was automated by leveraging the MS VOTT tool.
- The tagged frames were then used to train a model using CNTK and Faster RCNN. This model was then tested against more frames extracted from the videos. While this solution was good, it lacked speed and real-time video detection capabilities.
- As an enhancement to the solution, we moved to video object detection using YOLO V3, which provides a faster solution with real-time capabilities.
QUANTIFIABLE RESULTS FROM THE INTERVENTION
This web-based AI solution would save the client, valuable hours of expert biologist time and infrastructure costs spent in manually reviewing the videos. As part of a planned upgrade, an enhanced version of the solution has been provided to the customer, which predicts to deliver substantial cost savings to the tune of 80%.
OUR WORK MENTIONED IN SCIENCE JOURNALS
- Elsevier: MSR-YOLO: Method to Enhance Fish Detection and Tracking in Fish Farms
- Georgia State University Scholarworks: Technology Enabled Social Responsibility Projects and an Empirical Test of CSR’s Impact on Firm Performance
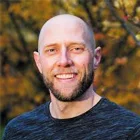
This project by Gramener is a good showcase of an AI-driven solution that addresses challenges faced by the Nisqually River Foundation.
Lucas Joppa,
Chief Environmental Officer Microsoft AI for EarthTHE IMPACT
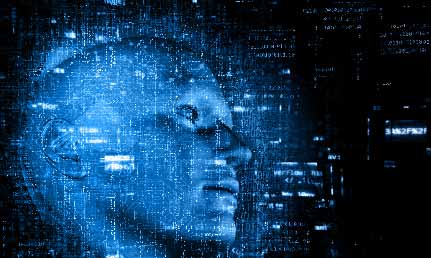
The AI-driven image recognition solution has led to potentially saving 80% of costs.
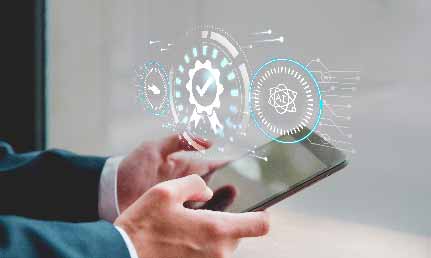
The automated solution has also reduced the time taken to perform the species detection task by 5X.
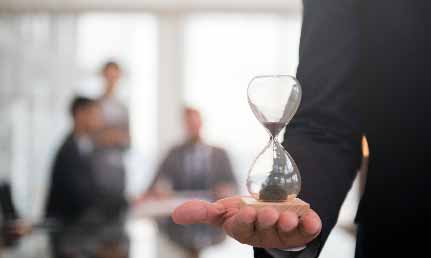
The solution helps save the time and efforts of expert biologists who would perform this task manually earlier.
Read more case studies
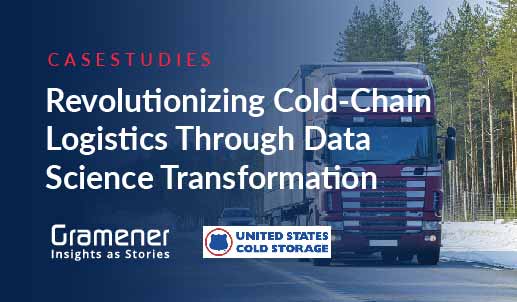
Revolutionizing Cold-Chain Logistics with Data Science
Gramener helped logistics leader United States Cold Storage revolutionize its logistics operations with a unique 'advisory-to-implementation’ approach.
Read More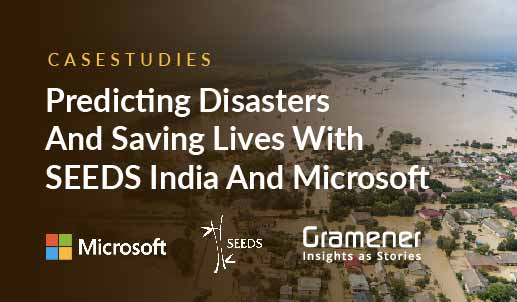
Predicting Disasters with SEEDS India and Microsoft
Gramener, in partnership with Microsoft, developed a Machine Learning – driven disaster impact model to predict disasters and save lives.
Read More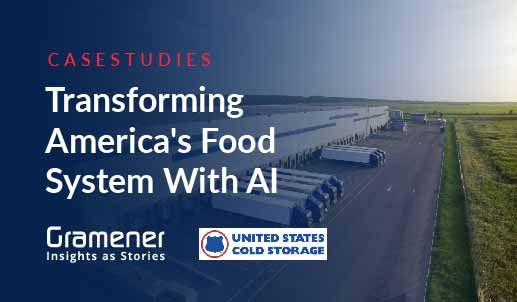
Transforming America's Food System using AI with USCS
We joined hands with USCS, a major in cold chain industry, to build an Intelligent Appointment Scheduler to automate the manual carrier scheduling.
Read More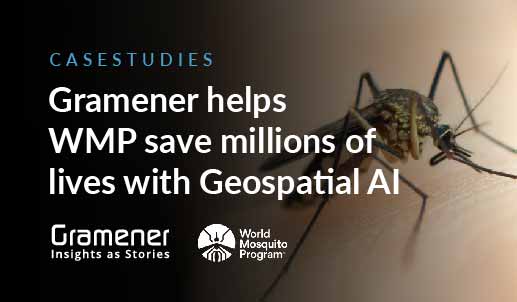
Fighting Diseases with Geospatial AI
Read and download the case study of our partnership with Microsoft and World Mosquito Program (WMP) to build Geospatial AI driven solution to fight mosquito borne diseases.
Read More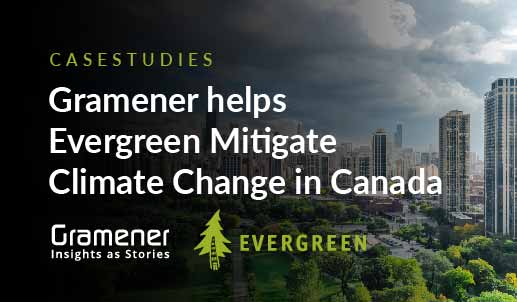
Building Urban Resilience with Spatial Analytics
Our partnership with Microsoft and Evergreen Canada resulted in building urban resilience by analyzing climate change & other datasets & building a unique Data Visualization tool.
Read More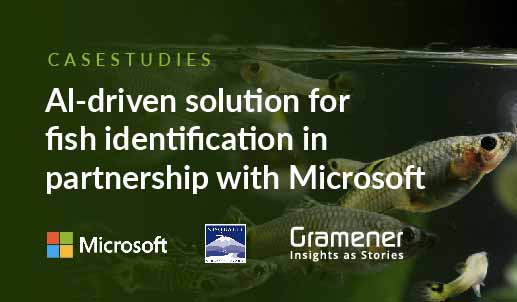
Salmon Detection Web App For Nisqually River Foundation
Read and download the case study of our partnership with Microsoft to develop AI solutions to automate fish species identification at the Nisqually River Foundation.
Read More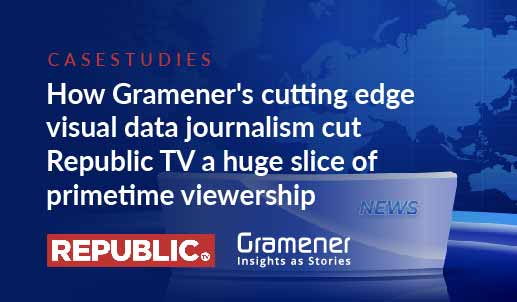
Primetime Viewership using Visual Data Journalism with Republic TV
Read and download the case study to know how Republic TV simplified election data reporting using data journalism and a cutting-edge visual analytics tool from Gramener.
Read More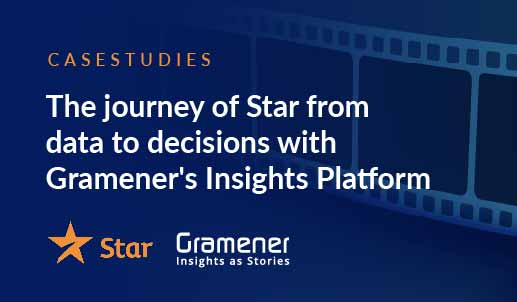
Automated Insights from Viewership Insights Platform
Read and download the case study to know how Star India is leveraging the Insights platform to understand their viewership across regions and create better content.
Read More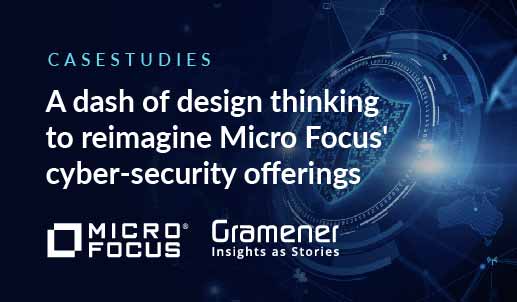
Data-Driven Design Thinking with Micro Focus
Read and download the case study to know how Micro Focus pitched its cybersecurity solution to a competitive market with data-driven design and storytelling features.
Read More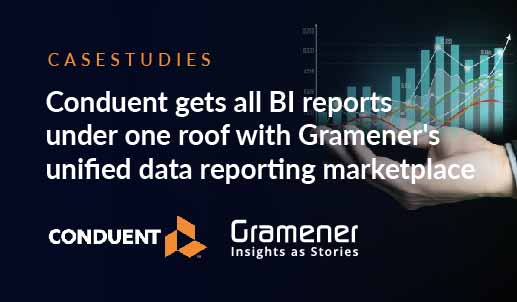
BI Reporting with Unified Data Marketplace For Conduent
Read and download the case study to know how Conduent is using a unified data marketplace to collaborate all BI reports at one place, enabling quick decision-making.
Read More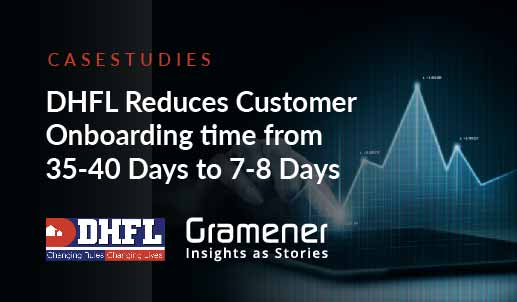
Visual Analytics Platform For Operational Excellence At DHFL
Read and download the case study to know how DHFL was able to sell their retail portfolio worth 15 thousand crores INR to nine banks using a visual analytics platform.
Read MoreGet a Copy of the case study
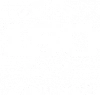
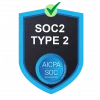
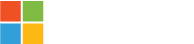