Predicting Disasters and Saving Lives
With Seeds and Microsoft
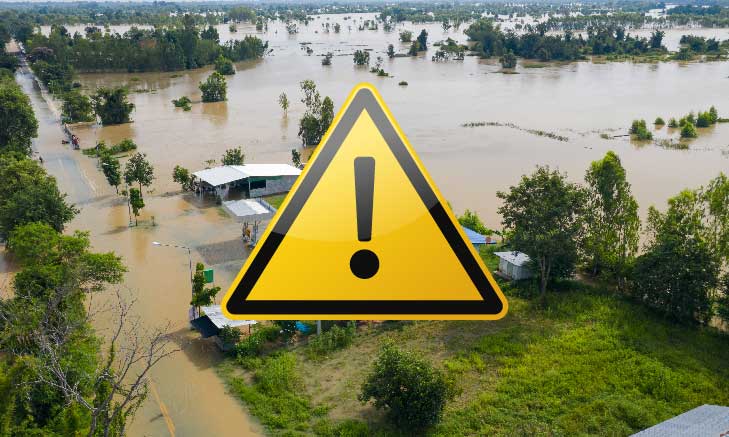
Challenge
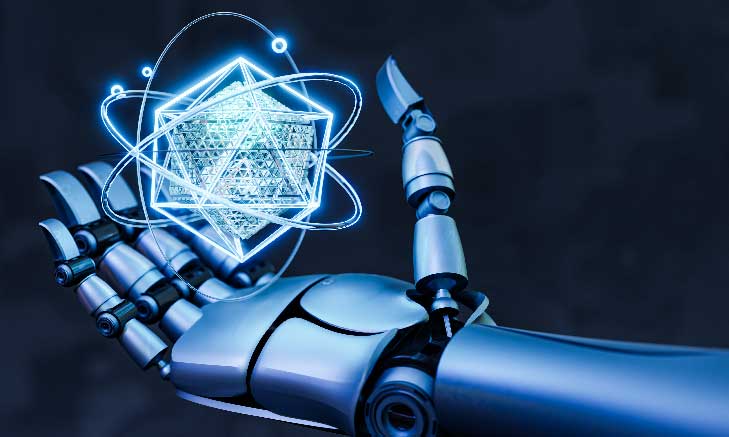
Approach
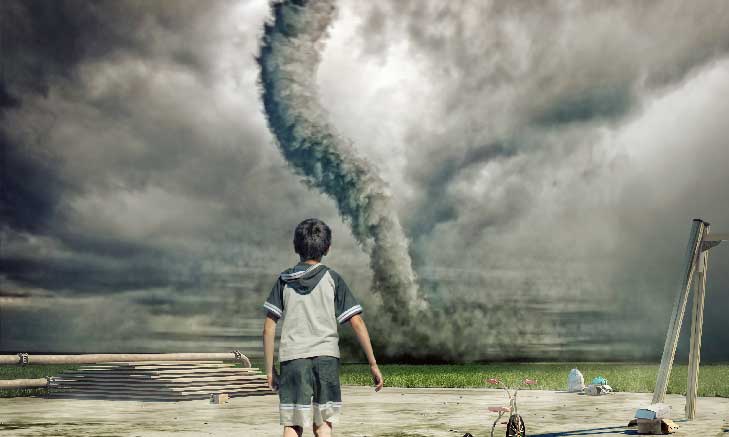
Outcome
Partnership - seeds & gramener join hands
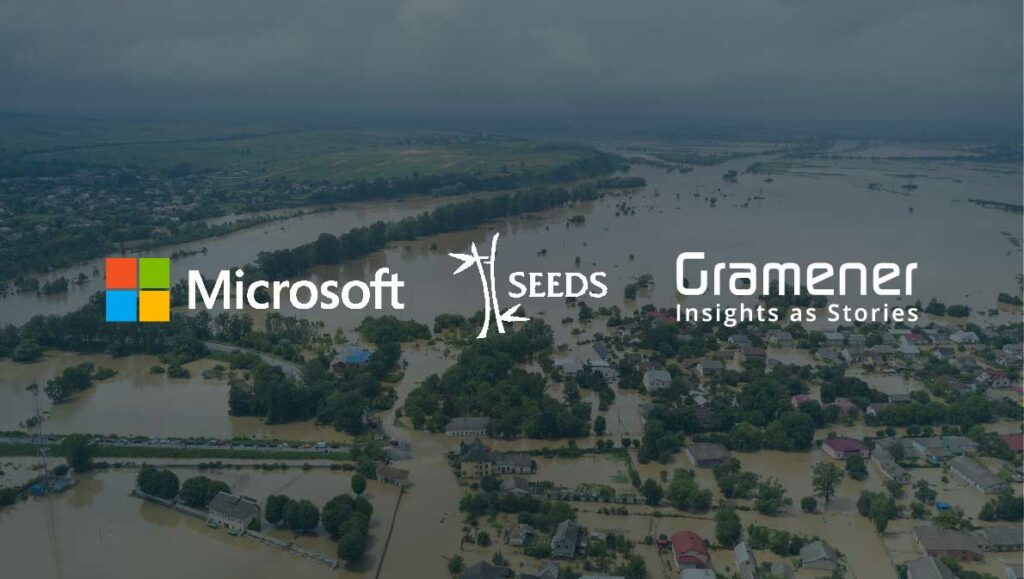
SEEDS is a not-for-profit organization that helps make communities resilient through comprehensive interventions in the areas of disaster readiness, response, and rehabilitation.
Gramener has partnered with SEEDS and Microsoft to develop the Sunny Lives AI model under Microsoft’s global program ‘AI for Humanitarian Action.
This predictive model aids in planning better risk reduction strategies against worsening climate emergencies and disasters.
Technology used for this solution
Creating the Sunny Lives model needed work from scratch due to some challenges:
- The population in those areas was dense enough to make accurate differentiation between houses difficult. As geographies change, so do the roof types, and identifying these different roof types was of prime importance for the model’s concept.
- There was no readily available training data to tag roof types for forecasting damage intensity.
Gramener, in partnership with Microsoft, built a Machine Learning model to counter these issues. To create the training data, Gramener accessed high-resolution satellite imagery and manually tagged over 50,000 houses. The roofs were classified under 7 categories based on the material used for their construction.
The final model could identify roofs with up to 90% accuracy. On top of this, Gramener added other geographic layers or parameters to the model.
The following parameters were considered – Waterbodies – River, Lake, Pond, and Sea, Distances from Road Network, Topographic Wetness Index (TWI), Elevation and Slope Vegetation (NDVI) – Sparse, Moderate, and Dense, Impervious surface, Landslide Risk, Building Footprints.
Developing the model took about 4 months. It was piloted in 2020 during cyclone Nivar and Burevi that hit the southern Indian states of Tamil Nadu and Kerala, respectively. The solution was deployed at scale in Puri during Cyclone Yaas in May 2021.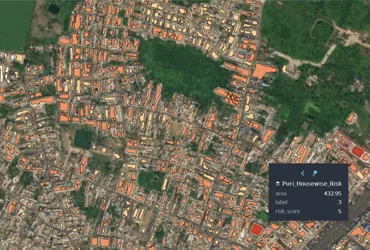
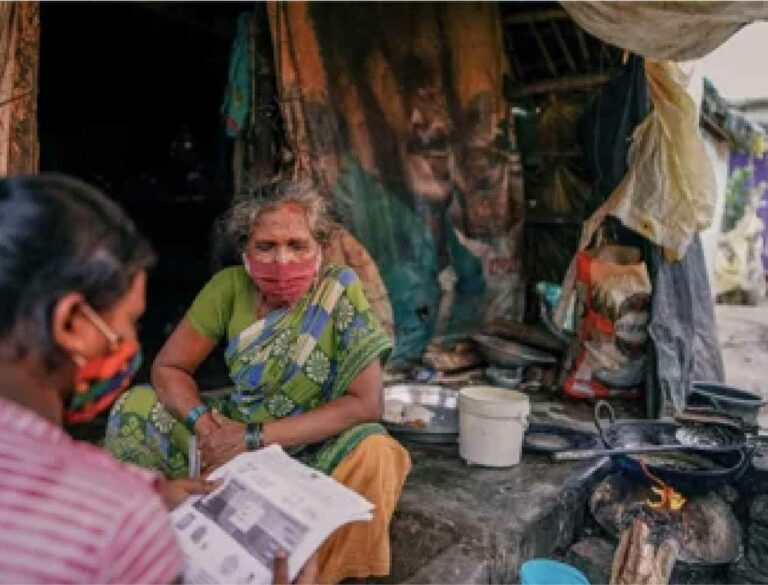
The solution required using a technology that could provide information that can be easily comprehended by the disaster response teams and the affected communities.
Considering that many disaster-prone areas are off the technology grid with low mobile penetration and network connectivity, it was necessary to break down the output from complex technologies like machine learning and artificial intelligence into simple and widely understood information. For example, SEEDS created specific advisories for dwelling types, suggesting the course of action for the individuals to reduce the risks.
A mock-up of the advisory shared by SEEDS with at-risk families. The solution utilizes high-resolution geospatial imagery and open datasets. It also uses geospatial analytics tools such as Kepler and Microsoft Azure to host and represent the results.
THE PROBLEM
When disasters like floods and heatwaves occur, warnings and other risk-related information are often vague and not up to date. Much of the current risk information work at a macro-level, covering hundreds of square meters of area and being too hard to understand by at-risk populations.
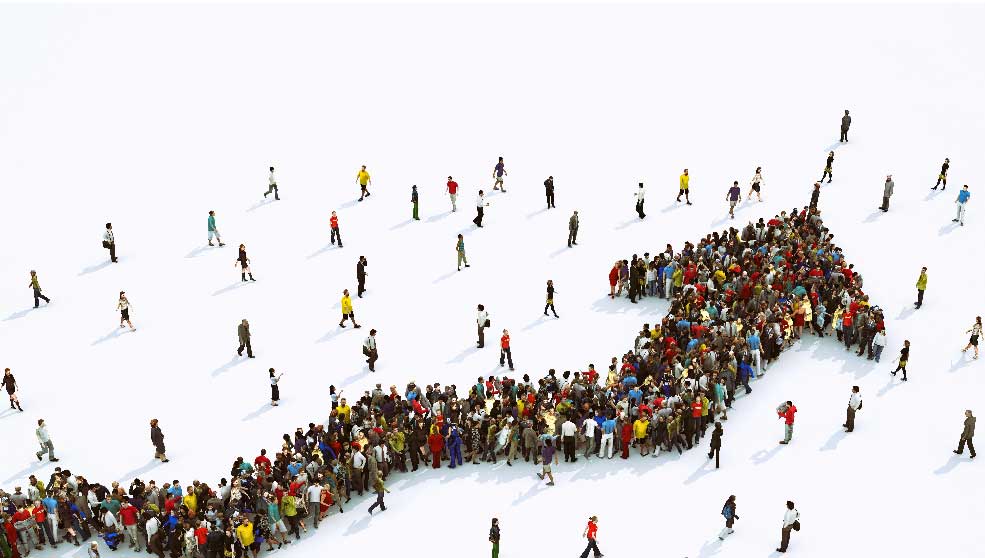
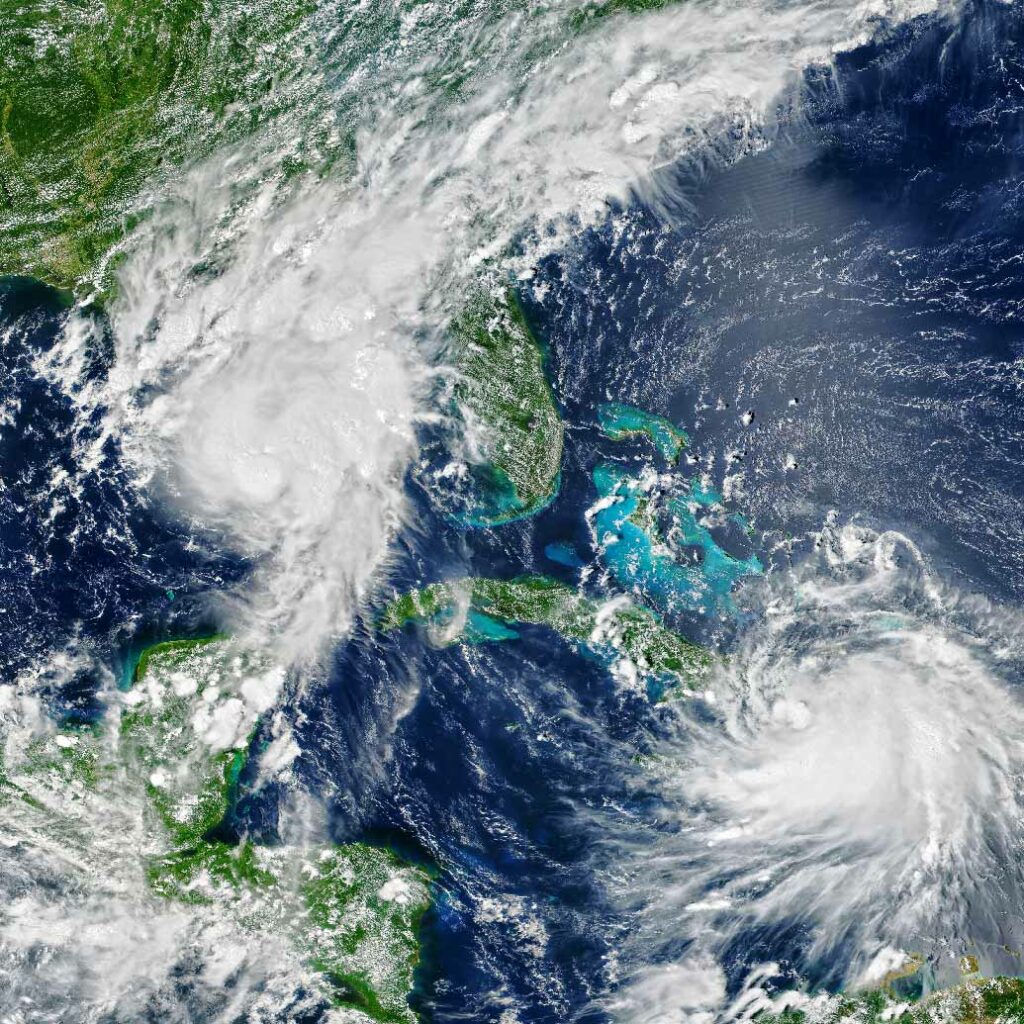
THE APPROACH
SEEDS partnered with Microsoft and Gramener to create an advanced machine learning-based model that could help them plan and respond more effectively to disasters.
This model uses historical data and satellite imagery to predict hyper-local risk information for early intervention. The Sunny Lives model builds on the knowledge that SEEDS has garnered over the years. The underpinning logic of the model is that the roofing material of a house can act as a proxy for its socio-economic condition. Hence, the capacity of a family living in a makeshift metal sheet home to respond and recover would be considerably less than that of one living in a large house constructed of concrete.
When two such dwellings exist in the same area, the impact of destruction caused by a disaster is significantly different for both. The solution maps this information on satellite imagery and other geographic parameters – forming the backbone of this AI solution.
THE IMPACT
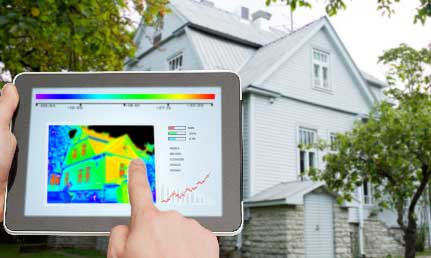
SEEDS could improve their dwelling detection rate from 52% to an impressive 88%
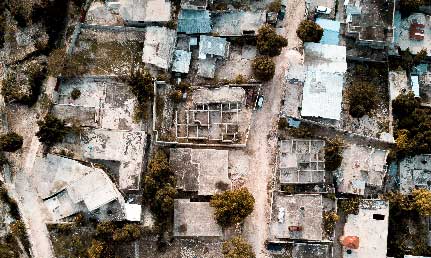
High impact assessment accuracy with >90% of damaged houses detected as high-risk
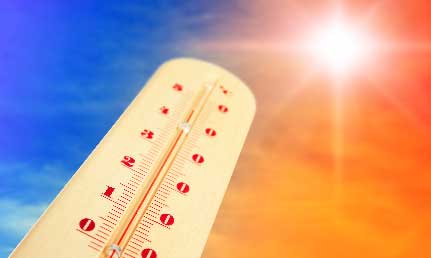
Model scaled for heatwaves sharing pre-emptive advisories to 50K individuals from at-risk communities
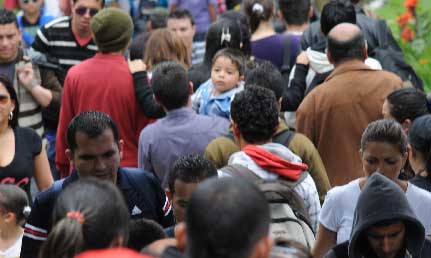
1.1K families were evacuated on time using the advisories generated by the model
Read more case studies
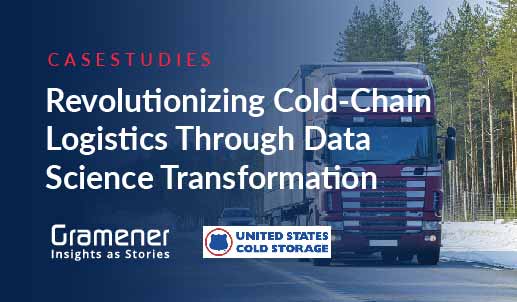
Revolutionizing Cold-Chain Logistics with Data Science
Gramener helped logistics leader United States Cold Storage revolutionize its logistics operations with a unique 'advisory-to-implementation’ approach.
Read More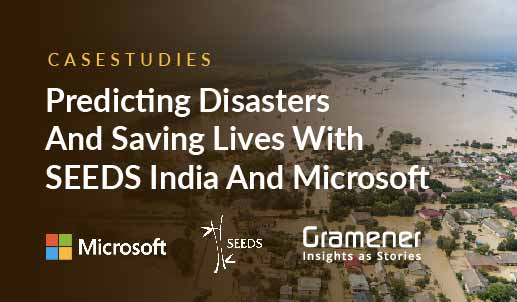
Predicting Disasters with SEEDS India and Microsoft
Gramener, in partnership with Microsoft, developed a Machine Learning – driven disaster impact model to predict disasters and save lives.
Read More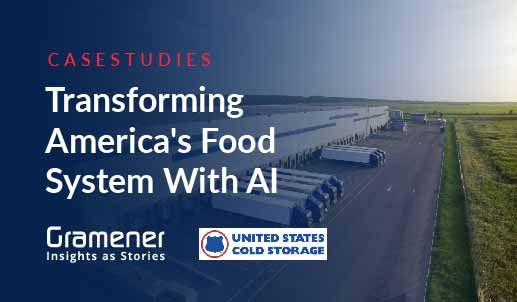
Transforming America's Food System using AI with USCS
We joined hands with USCS, a major in cold chain industry, to build an Intelligent Appointment Scheduler to automate the manual carrier scheduling.
Read More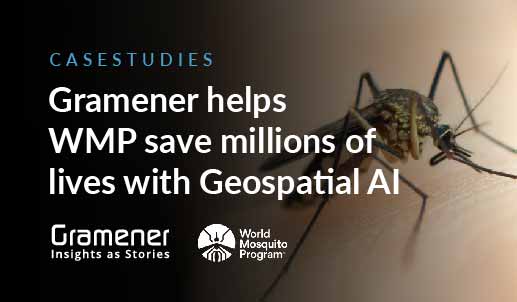
Fighting Diseases with Geospatial AI
Read and download the case study of our partnership with Microsoft and World Mosquito Program (WMP) to build Geospatial AI driven solution to fight mosquito borne diseases.
Read More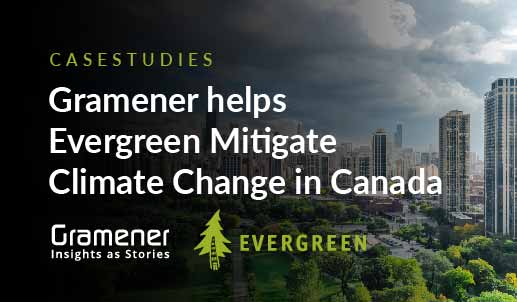
Building Urban Resilience with Spatial Analytics
Our partnership with Microsoft and Evergreen Canada resulted in building urban resilience by analyzing climate change & other datasets & building a unique Data Visualization tool.
Read More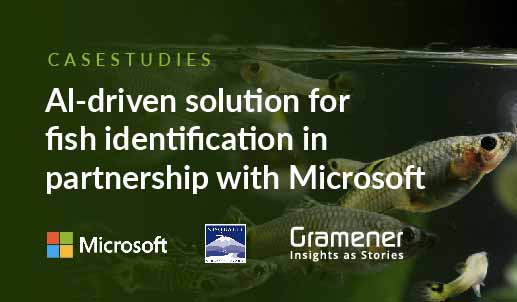
Salmon Detection Web App For Nisqually River Foundation
Read and download the case study of our partnership with Microsoft to develop AI solutions to automate fish species identification at the Nisqually River Foundation.
Read More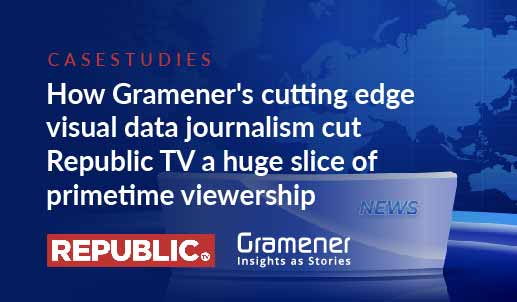
Primetime Viewership using Visual Data Journalism with Republic TV
Read and download the case study to know how Republic TV simplified election data reporting using data journalism and a cutting-edge visual analytics tool from Gramener.
Read More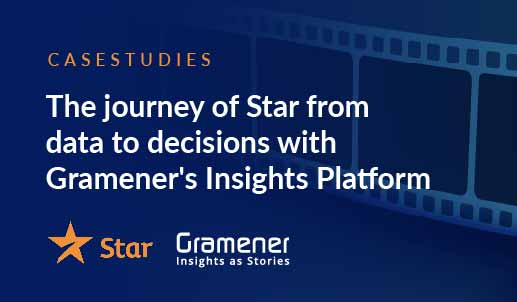
Automated Insights from Viewership Insights Platform
Read and download the case study to know how Star India is leveraging the Insights platform to understand their viewership across regions and create better content.
Read More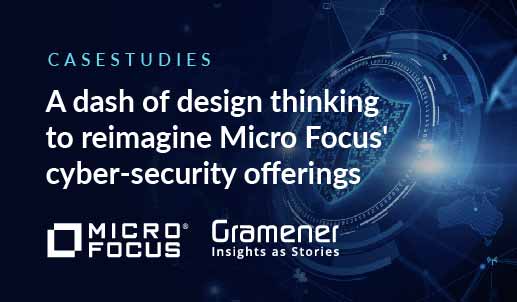
Data-Driven Design Thinking with Micro Focus
Read and download the case study to know how Micro Focus pitched its cybersecurity solution to a competitive market with data-driven design and storytelling features.
Read More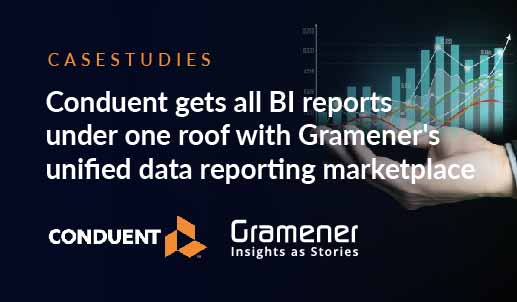
BI Reporting with Unified Data Marketplace For Conduent
Read and download the case study to know how Conduent is using a unified data marketplace to collaborate all BI reports at one place, enabling quick decision-making.
Read More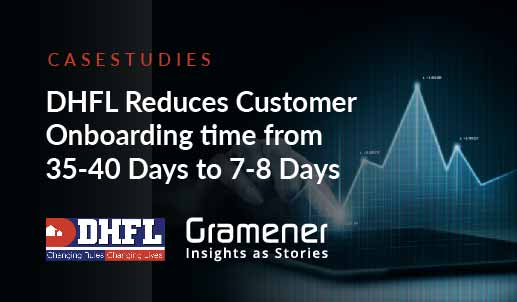
Visual Analytics Platform For Operational Excellence At DHFL
Read and download the case study to know how DHFL was able to sell their retail portfolio worth 15 thousand crores INR to nine banks using a visual analytics platform.
Read MoreGet a Copy of the case study
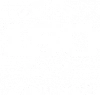
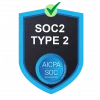
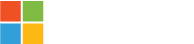